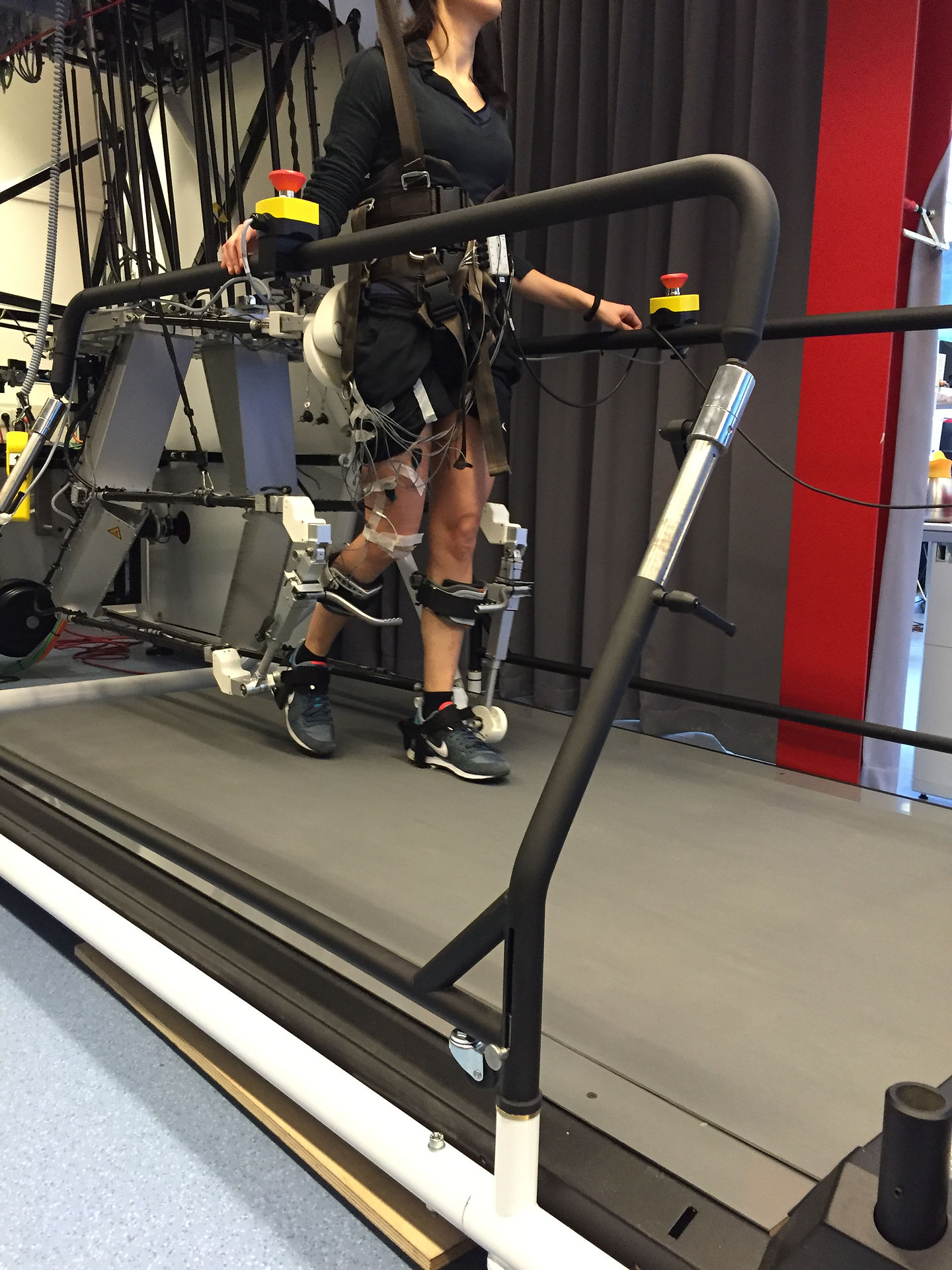
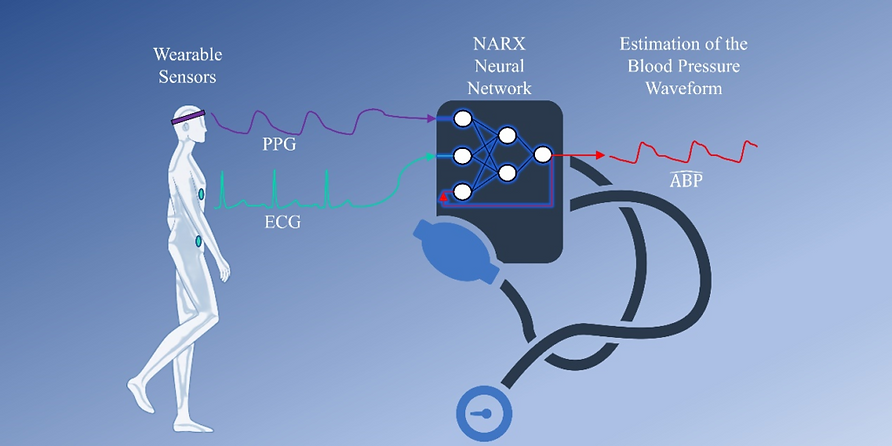
Biomedical Sensors and Predictive Modeling
Development of Sensors and Data-driven Techniques to Address the Needs of Clinicians.
This includes
1) Machine Learning techniques for accurate and reliable cuffless blood pressure estimation for bedridden individuals and during the normal activity of daily living as well as moderate to heavy exercise sessions
2) sensors and algorithms for accurate gait analysis
3) data-driven techniques for reliable prediction of freezing of gait in Parkinson's Disease
4) Smart implants and algorithm for endo-prosthetic joints monitoring

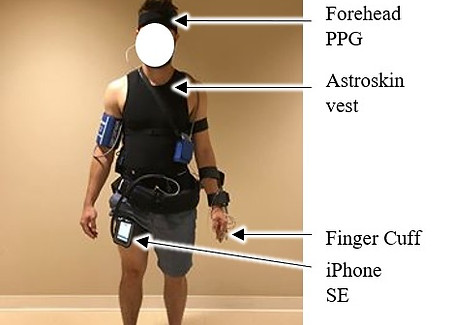
Blood Pressure and Blood Flow prediction and optimization
We are developing advanced machine learning techniques for reliable and accurate prediction of blood pressure both for long term cuffless blood pressure monitoring and optimizing the blood flow using a wearable compression device developed by Fluid Flow Physics Group at the University of Waterloo.
We used machine learning techniques to optimize the blood flow for each individual wearing the device.
BP nonlinear dynamic modeling (C. Landry et al., IEEE Sensors 2019)
Cuffless BP estimation during activity of daily living (C. Landry et al., IEEE JBHI 2021)
A fusion approach to improve BP accuracy and estimate its uncertainty (C. Landry et al., Scientific Reports 2022)
Cuffless BP during moderate and high intensity activities (C. Landry et al., IEEE JBHI 2022)
Investigating Optimal Intermittent Pneumatic Compression Timing (I. Santelices, et al., IEEE BSN 2023)
Prediction and Classification of Freezing of Gait in Parkinson's Disease
From developing new features that can be
used in detection and prediction of gait freezing
to development of data-driven probabilistic predictive models to predict freezing seconds before its occurrence.
Accurate and Reliable Freezing of Gait Prediction (A. Arami et al., IEEE TNSRE 2019)
Freezing of Gait Detection Feature Analysis (A. Arami et al., ISB 2019)
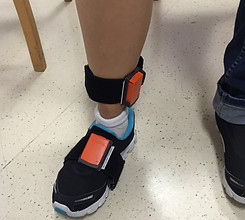

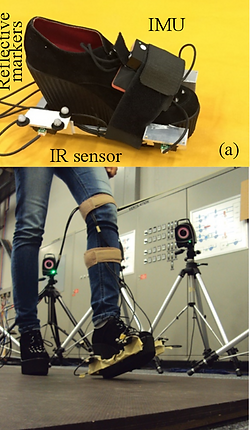
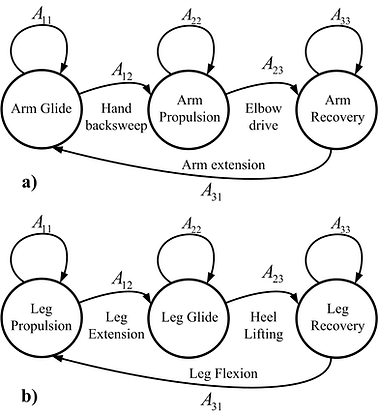
Sensors and Machine Learning For Accurate Activity Classification and Gait and Swimming Metric Estimation
The goal is to design implantable instrumentation to estimate clinically meaningful measures related to the function of a prosthetic joint. Variety of sensors for both kinematics estimation and joint contact forces have been used along with the data-driven models to provide an accurate estimate and diagnose any fault with the prostheses.
Online Foot Clearance Estimation (A. Arami, et al., IEEE Sensors 2017).
Improving Activity Recognition Using a Wearable Barometric Pressure Sensor in Mobility-impaired Stroke Patients (F. Massé, et al., J. NeuroEngineering and Rehabilitation2015).
A Hidden Markov Model of the Breaststroke Swimming Temporal Phases Using IMUs
(F. Dadashi et al., IEEE BSN 2013)

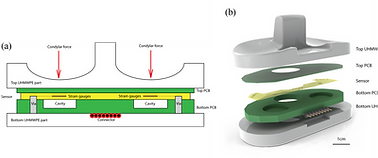

Smart Orthopedic Implant for Monitoring Function and Loosening Detection
The goal is to design implantable instrumentation to estimate clinically meaningful measures related to the function of a prosthetic joint. Variety of sensors for both kinematics estimation and joint contact forces have been used along with the data-driven models to provide an accurate estimate and diagnose any fault with the prostheses.
Knee Implant Loosening Detection (A. Arami, et al., Annals of Biomedical Engineering 2018).
Personalized Modeling for Patellar Implant Resurfacing (A. Latypova, et al., Clinical Biomechanics 2016).
Implantable Knee Contact Force Sensors (gen. 2) (D. Forchelet, et al., Sensors 2014).
Knee-Implant-Embedded Magnetic Sensors Calibration (A. Arami, et al., IEEE Sensors 2014).
Knee implant force sensors (gen. 1) (W. Hasenkamp, et al., Medical Engineering and Physics 2014).
Concurrent STA-free system for knee concurrent rotations (A. Arami, et al, IEEE Trans. Biomed. Eng. 2013).
Smart Implant for Knee function monitoring (A. Arami et al., IEEE Trans. Autom. Sci. and Eng. 2013).